This blog was written by Antoinette Bhattacharya from the IDEAS team and first published on the HNN blog.
Working with the government of Gombe, we investigated the quality of routine data from the District Health Information Software 2 (DHIS 2), an open source information system used in over 60 low- and middle-income countries.
What did we do?
First, we studied the monitoring frameworks of the Ending Preventable Maternal Mortality and Every Newborn Action Plan strategy documents to identify priority indicators. These were then mapped to the data that facilities in Gombe State were already documenting and reporting on, such as whether women were receiving a uterotonic to prevent postpartum haemorrhage or whether newborns were immediately receiving care to keep warm after delivery.
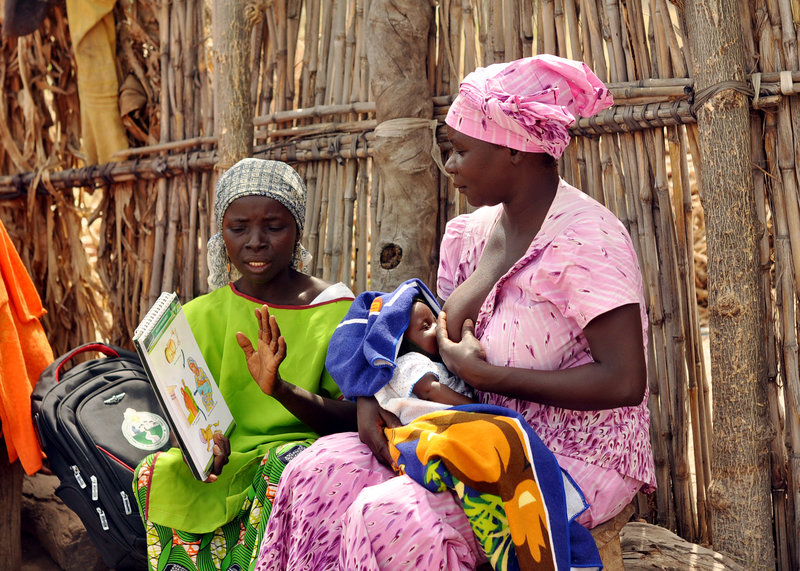
Once the indicators were identified, we assessed their quality in DHIS 2, according to the World Health Organization’s Data quality review: a toolkit for facility data quality assessment. We reviewed three overarching data quality dimensions:
• Completeness and timeliness
• Internal consistency (consistency between indicators with a predictable relationship, trends over time, outliers, agreement between facility records and other data sources)
• External consistency
What did we find?
We identified 14 indicators that could be tracked through facility-based data; these indicators monitor routine care that all women and newborns should receive during facility visits. Of these, 12 indicators were included in Gombe’s DHIS2.
When we reviewed the data quality for July 2016-June 2017, we found that facility-reported data in DHIS 2 were incomplete at least 40% of the time, under-reported 10%-60% of the events documented in the facility registers, and showed inconsistencies over time, between indicators with a predictable relationship, and with external data sources such as household surveys.
Positively, the quality of the indicators was not universally nor equally poor. There were differences in data quality by indicator type. For example, contact indicators had higher overall data quality than indicators related to the provision of commodities. Indicators for content of care had the weakest quality.
What does this mean?
This study emphasizes the need for coordinated action at all levels of the health system to ensure good data quality for monitoring.
First, it is essential to strengthen what is already there to rationalize and maximize completeness of existing data. For example, in Gombe State, there were priority indicators being documented by facility staff, such as essential newborn care, that weren’t being reported for additional monitoring at the district and national levels. Also, there were indicators that had to be recorded in multiple registers, which not only involved extra work, but also promoted confusion when those sources didn’t agree.
Second, an investment in DHIS 2 should include ongoing reviews of its content and structure to promote data quality and fitness for purpose. For Gombe State, the maternal and newborn health data in DHIS 2 could not be used “off the shelf.” It wasn’t possible to distinguish between missing and true zero values, an important consideration when assessing an indicator’s completeness. Also, extra preparation was necessary to identify and delete inactive facilities, inactive sub-districts, duplicate facilities, and duplicate indicators.
Lastly, it is important to routinize data quality review, feedback, and supervision. Even technology-based innovations like DHIS 2 need feedback and supervision to realize the potential of using routine data for improving the health and survival of women and newborns.